.jpg)
.jpg)
Introduction
Predictive maintenance systems have shown great potential in identifying early-stage motor issues autonomously. However, most existing predictive maintenance systems rely on supervised learning, which necessitates time-consuming manual data collection and annotations. Moreover, these systems are typically designed to address single motor issues and struggle to adapt to varying motor speed and load conditions. Consequently, they are not practical for industrial applications.
To address these limitations, ITR introduces Advancing Predictive Maintenance (APM) systems, an innovative unsupervised anomaly detection system for electric motors.
Challenges faced by Predictive Maintenance
The manufacturing industry faces a significant challenge as electric motors operate continuously 24/7, leading to the development of various defects. If left untreated, these issues can result in machine breakdowns, production downtime, and financial losses. To address this critical problem, there is an increasing demand for predictive maintenance systems for motors.
Traditional maintenance methods involve manual SCADA systems with hardcoded thresholds and static alert rules. However, these approaches overlook the dynamic behavior of machinery and fail to consider contextual data, leading to potential false alarms.
Unlike traditional methods, predictive maintenance systems are less exhaustive, more accurate, and have the potential for autonomous operation. However, most available predictive maintenance systems require real-time data collection and manual annotations to train fault prediction models, which can be time-consuming for companies. Additionally, these systems typically focus on addressing a single type of motor fault, disregarding the possibility of multiple faults like bearing faults, rotor faults, and stator issues that can occur in electric motors.
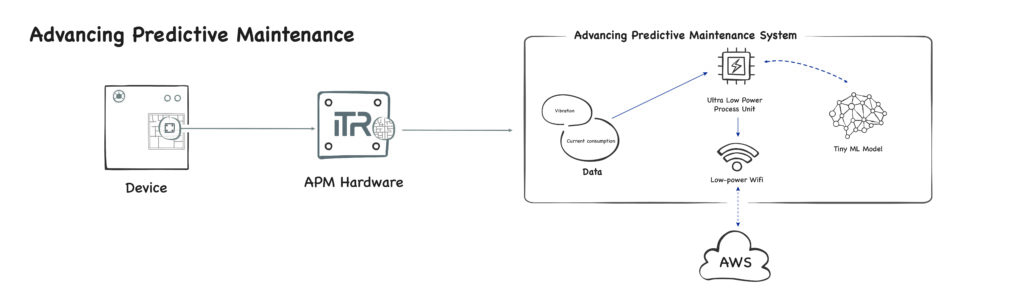
ITR’s Solutions
Advancing Predictive Maintenance (APM) systems utilize vibration and current consumption data to detect anomalies, effectively capturing various motor defects. With a range of advanced features, customers can effortlessly collect data, train models, and deploy them autonomously. The system is highly implementable and enables customers to remotely monitor the motor’s condition, ensuring seamless tracking of its performance.
To address the market needs, ITR has incorporated Tiny Machine Learning (TinyML) into the development of their algorithms. This allows the anomaly detection algorithms to run directly on single board computers, which can be attached directly to the motors of machines. ITR’s system eliminates the need for streaming sensor data to the cloud, avoiding bandwidth waste and the strain on storage resources and server processing capabilities. Customers can simply deploy this system using Serverless services.
Conclusion
Predictive maintenance is essential in the manufacturing industry as it reduces downtime and maintenance costs by preventing sudden breakdowns. However, as factories become more complex, the number of motors to be monitored increases, leading to a larger volume of data to process. Sending all this data to the cloud for processing is not feasible due to bandwidth limitations, latency issues, and cost constraints. Previous approaches have focused on deep learning-driven onboard anomaly detection, but they require expensive and power-hungry hardware with high computational resources.
Fortunately, recent advancements in Tiny Machine Learning enable the design and deployment of deep neural networks on low-cost, resource-constrained embedded hardware. In this case study, we propose an predictive maintenance using Autoencoder reconstruction and anomaly detection in time-series vibration sensor data. The designed Auto-Encoder model has a footprint of 22 KB and was deployed on a resource-constrained ARM Cortex-M4 microcontroller with 256KB of SRAM and 1MB Flash.
The model was validated using previous machine data and achieved an accuracy and precision of approximately 82%. Currently, by using post-training quantization, we are trading off the model’s accuracy to reduce its size. In the future, we plan to employ Quantization-Aware Training, which will help us achieve even higher model accuracy.